top of page
Search
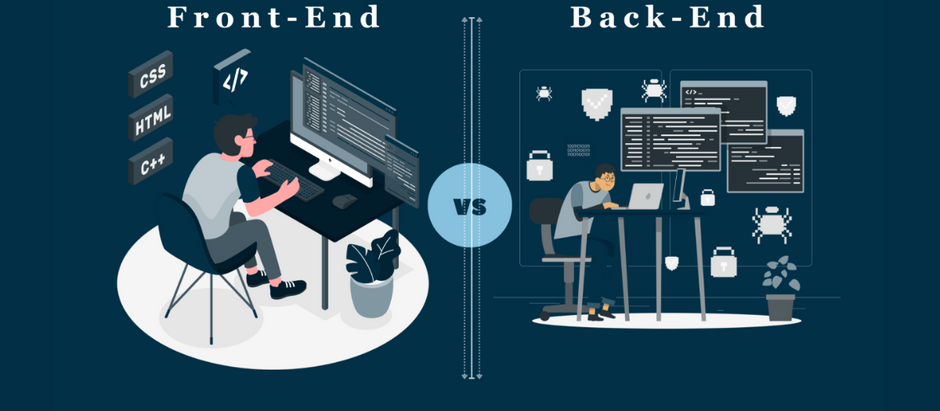
archi jain
Dec 12, 20245 min read
Building Powerful Websites: A Journey from Frontend to Backend
Creating a powerful website is like constructing a building. It involves many layers, from designing the user interface to ensuring that...
2 views0 comments

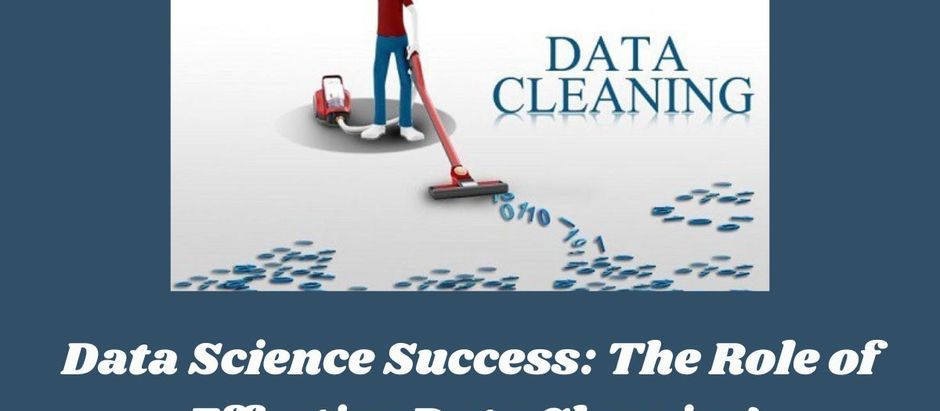
archi jain
Dec 9, 20245 min read
Data Science Success: The Role of Effective Data Cleaning
In the world of data science, the quality of your data is crucial for making informed, accurate decisions. Data cleaning, or data...
2 views0 comments
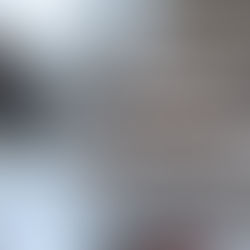
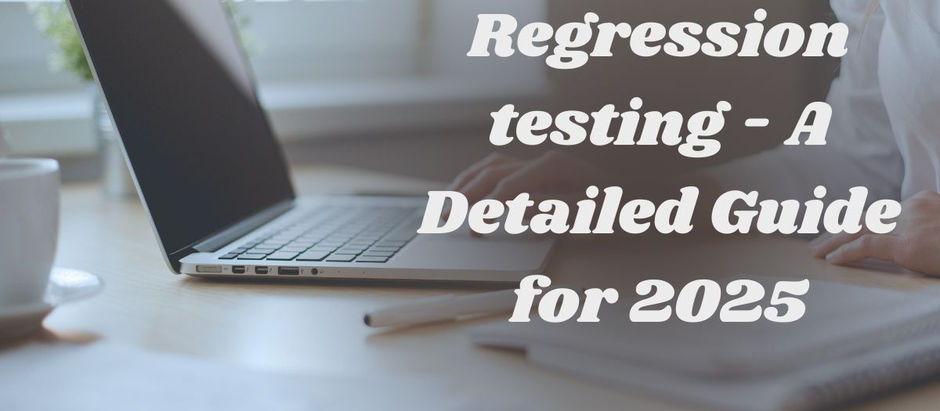
archi jain
Dec 7, 20245 min read
Regression testing - A Detailed Guide for 2025
In the world of software development, ensuring that a product works as expected after changes are made is crucial. This is where...
3 views0 comments
bottom of page