top of page
Search

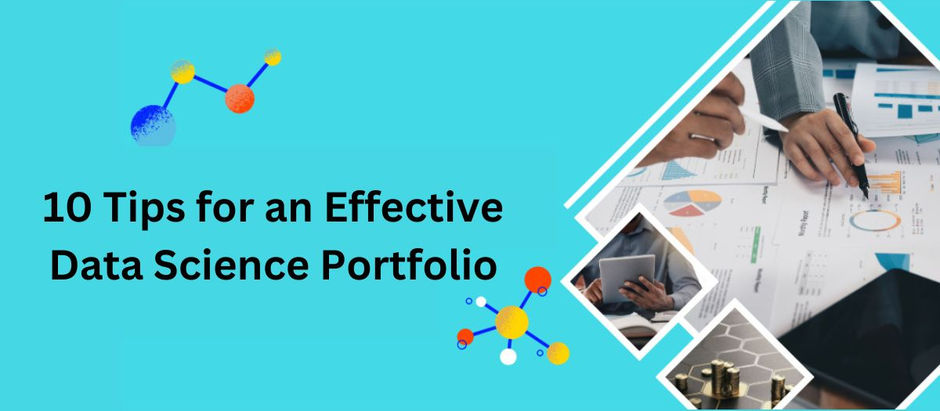
10 Tips for an Effective Data Science Portfolio
Creating an effective data science portfolio is a key step toward showcasing your skills and landing a job in this competitive field....
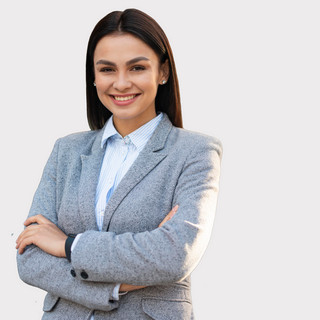
archi jain
Dec 6, 20244 min read
3 views
0 comments

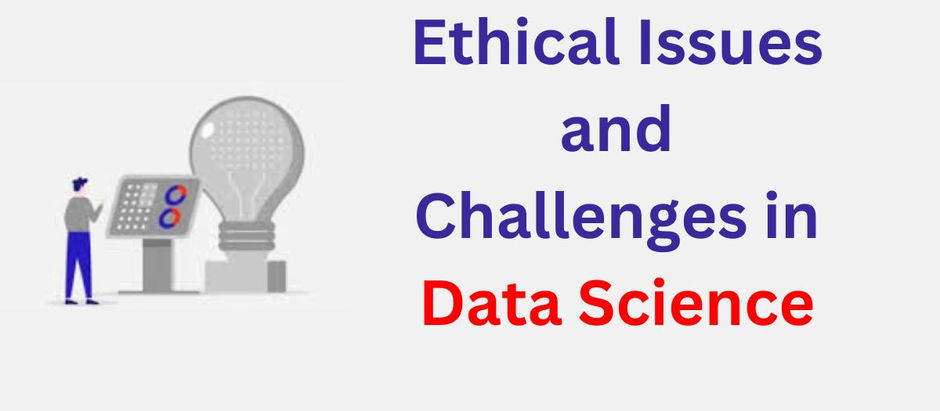
Ethical Issues and Challenges in Data Science
In the age of big data and advanced analytics, data science has become a powerful tool driving innovation, improving decision-making, and...
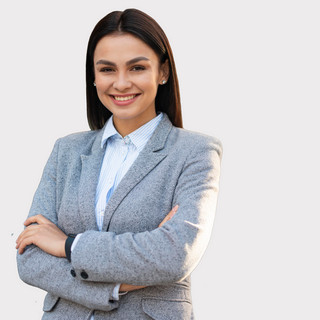
archi jain
Dec 5, 20245 min read
1 view
0 comments
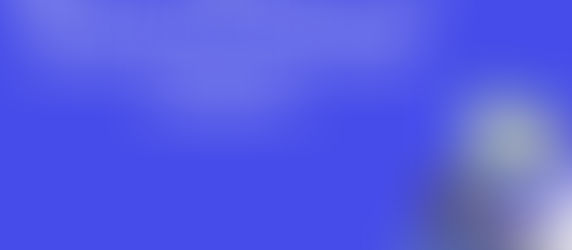
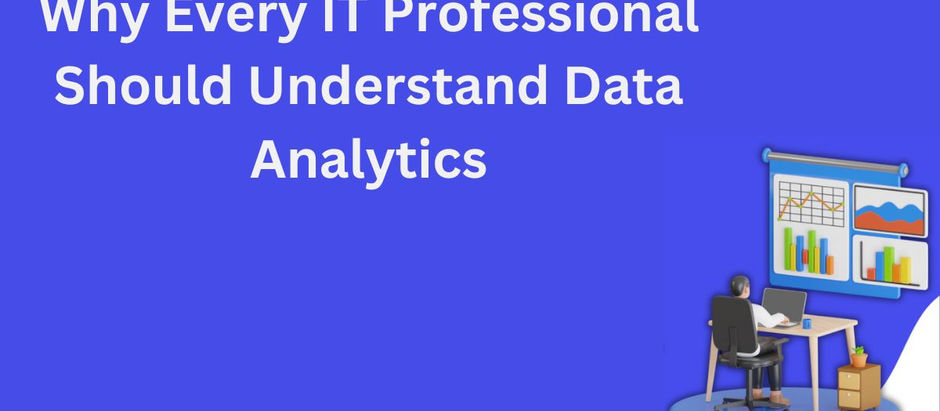
Why Every IT Professional Should Understand Data Analytics
Introduction In today’s tech-driven world, data is everywhere. From user interactions to system logs, businesses collect massive amounts...
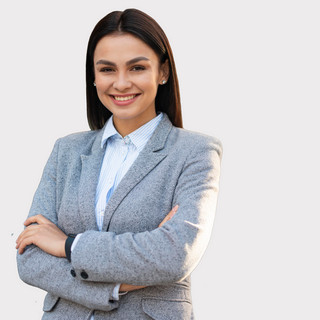
archi jain
Dec 4, 20245 min read
2 views
0 comments
bottom of page