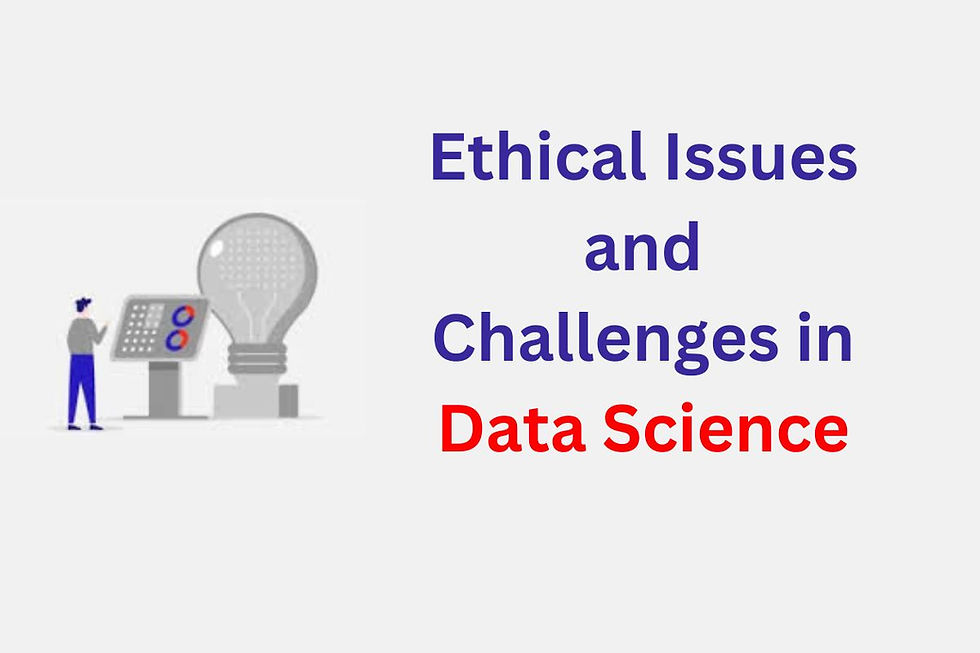
In the age of big data and advanced analytics, data science has become a powerful tool driving innovation, improving decision-making, and transforming industries. However, with its growing influence comes a host of ethical issues and challenges that require careful consideration. Data scientists must not only focus on technical skills but also on the ethical implications of their work to ensure fairness, transparency, privacy, and accountability.
Navigating the Ethical Challenges in Data Science
"Navigating the Ethical Challenges in Data Science" involves addressing key issues such as data privacy, bias, transparency, and accountability. Data scientists must ensure that their work upholds privacy rights, reduces bias, provides clear explanations for algorithmic decisions, and ensures informed consent, all while minimizing harm and promoting fairness.
Privacy Concerns and Data Protection
One of the most pressing ethical issues in data science is privacy. Data scientists often work with sensitive information, such as personal details, health records, and financial transactions. Collecting, storing, and analyzing this data raises serious concerns about how it is protected and whether it is being used responsibly.
Key Points:
Consent: Individuals should be informed about how their data will be used and have the option to opt-in or opt-out.
Data Minimization: Data should only be collected for the specific purpose it was intended for, avoiding unnecessary data collection.
Security: Measures must be in place to protect data from breaches or misuse, ensuring that only authorized individuals can access it.
Challenge: Balancing the need for data analysis with the rights of individuals can be difficult, especially when working with vast amounts of personal information.
Bias and Discrimination
Another significant ethical issue in data science is bias. Algorithms and models are only as good as the data fed into them. If the data reflects historical biases or is not representative, the resulting analysis or predictions may perpetuate inequality or unfair treatment.
Key Points:
Data Bias: Data may contain biases due to human errors, cultural stereotypes, or historical injustices.
Algorithmic Bias: Models trained on biased data may produce discriminatory outcomes, such as favoring one group over another in hiring, lending, or law enforcement.
Impact on Vulnerable Groups: Biased algorithms can disproportionately affect marginalized communities, exacerbating existing social inequalities.
Challenge: Ensuring that data is fair and unbiased requires constant vigilance, continuous testing, and improvements to both the data collection process and the algorithms used for analysis.
Transparency and Accountability
Data science often operates as a "black box," with complex algorithms making decisions that can be difficult to understand or explain. This lack of transparency can lead to mistrust, especially when decisions made by algorithms have real-world consequences, such as in criminal justice, healthcare, or hiring.
Key Points:
Explainability: Data scientists must strive to develop models that are interpretable and understandable to both experts and non-experts.
Accountability: When algorithms make mistakes or cause harm, it is crucial to determine who is responsible and how to correct the issue.
Trust: Transparent processes and accountability can build trust in data-driven decisions.
Challenge: Striving for transparency in highly complex models, such as deep learning, where interpretability is often limited, is a difficult task.
Data Ownership and Consent
Who owns the data collected, and how can it be used? This is a fundamental ethical issue in data science, particularly in industries like healthcare, where personal data is extremely sensitive. The concept of "data ownership" is not always clear, and questions about how individuals' data should be used or shared are ongoing.
Key Points:
Ownership: It’s essential to establish clear guidelines about who owns data—the individual, the company that collects it, or the platform that stores it.
Informed Consent: Individuals should be aware of and consent to how their data will be used, whether it’s for research, commercial purposes, or sharing with third parties.
Right to be Forgotten: In some cases, individuals may want to delete their data, which presents a challenge for companies that rely on that data for analysis.
Challenge: Ensuring that companies respect individuals' rights while balancing the need for data collection and analysis can be complicated.
Impact on Employment and Society
As data science becomes more integrated into automation and artificial intelligence (AI), there is growing concern about the displacement of jobs. Machines powered by AI are increasingly capable of performing tasks traditionally done by humans, leading to concerns about job loss and social inequality.
Key Points:
Job Displacement: Automation and AI may replace jobs in sectors like manufacturing, transportation, and even services like customer support.
Reskilling and Education: Data scientists, policymakers, and organizations must work together to provide opportunities for workers to learn new skills and transition to new roles.
Social Impact: The widespread use of AI and automation could lead to economic inequality if the benefits are not widely distributed.
Challenge: Balancing the advancements in data science with the potential societal impacts requires careful policy planning and a commitment to ethical principles that prioritize human well-being.
Data Misuse and Manipulation
Data science is often used to influence opinions, behaviors, and decision-making. Whether it’s through targeted political ads, personalized marketing, or media manipulation, there is a growing concern about the ethical implications of using data to manipulate individuals or groups.
Key Points:
Misinformation: Data can be used to spread false or misleading information, especially when it comes to politics or social issues.
Manipulation: Personal data can be exploited to influence people’s choices in ways that may not be in their best interest.
Consumer Protection: People should be aware of how their data is used for marketing purposes, and there should be safeguards to prevent deceptive practices.
Challenge: While data can be a powerful tool for improving decision-making, it also has the potential to be misused. Ensuring that data is used ethically to benefit society is a critical responsibility for data scientists.
Ethical Decision-Making in Algorithm Development
Data scientists are responsible for the ethical implications of the algorithms they develop. These algorithms, which drive everything from recommendation systems to autonomous vehicles, must be designed with ethical considerations in mind to avoid unintended harm.
Key Points:
Fairness: Data scientists must ensure that algorithms treat all users or subjects fairly, without discrimination.
Safety and Security: Algorithms, particularly those used in autonomous systems, must be designed to prioritize safety and prevent harm.
Ethical Guidelines: It’s important for data scientists to adhere to ethical guidelines and frameworks, which can help guide their decisions and actions.
Challenge: Ethical decision-making is not always straightforward, and balancing technical objectives with moral considerations requires ongoing reflection and evaluation.
Conclusion
Data science is a transformative field with the potential to greatly benefit society, but it also presents numerous ethical challenges. Privacy, bias, transparency, accountability, and the impact on employment are just a few of the ethical issues that data scientists must navigate. By being aware of these challenges and incorporating ethical principles into their work, data scientists can help ensure that their contributions are not only technically effective but also socially responsible and fair.
The responsibility lies with data scientists, organizations, and policymakers to create frameworks and policies that protect individuals, promote fairness, and encourage transparency. For those interested in pursuing a career in data science, taking a Data Science course in Hyderabad, Delhi, Gurgaon, and other locations in India can provide the skills necessary to tackle these ethical dilemmas. With thoughtful approaches and a commitment to ethics, data science can continue to thrive while minimizing its risks and maximizing its benefits for society.
Comentários