Key Methods for Hypothesis Testing: The Top 10 Techniques
- archi jain
- Jul 13, 2024
- 3 min read
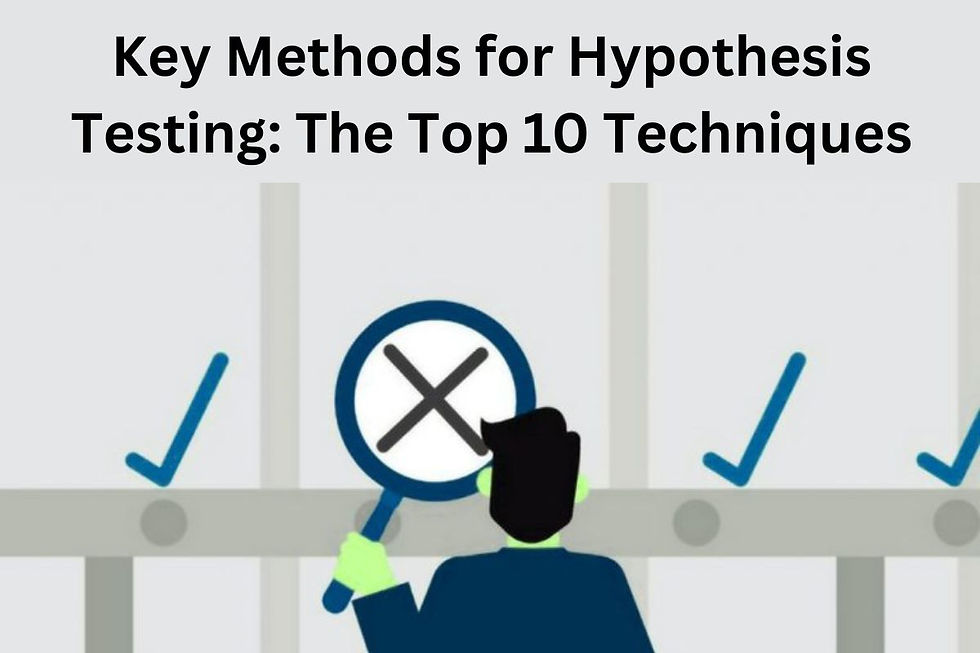
Hypothesis testing is a cornerstone of statistical analysis, playing a crucial role in scientific research, business decision-making, and various fields where data-driven insights are essential. At its core, hypothesis testing involves making an assumption (the hypothesis) about a population parameter and then using sample data to test the validity of that assumption. By systematically evaluating evidence through hypothesis tests, researchers can make informed conclusions about whether observed patterns in data are due to chance or represent genuine effects.
Top 10 techniques used for hypothesis testing
1. Z-Test
The Z-test is used when the population variance is known and the sample size is large (usually n > 30). It determines whether there is a significant difference between the sample mean and the population mean.
Null Hypothesis (H₀): The sample mean is equal to the population mean.
Alternative Hypothesis (H₁): The sample mean is not equal to the population mean.
2. T-Test
The T-test is used when the population variance is unknown and the sample size is small (usually n < 30). There are different types of T-tests: one-sample, independent two-sample, and paired sample T-tests.
One-Sample T-Test: Tests if the sample mean is equal to a known value.
Independent Two-Sample T-Test: Compares the means of two independent groups.
Paired Sample T-Test: Compares means from the same group at different times.
3. Chi-Square Test
The Chi-Square test is used for categorical data to assess how likely it is that an observed distribution is due to chance. There are two main types: the Chi-Square goodness of fit test and the Chi-Square test for independence.
Goodness of Fit: Tests if a sample matches a population.
Test for Independence: Tests if two categorical variables are independent.
4. ANOVA (Analysis of Variance)
ANOVA tests the difference between the means of three or more groups. It determines if at least one group mean is different from the others.
Null Hypothesis (H₀): All group means are equal.
Alternative Hypothesis (H₁): At least one group mean is different.
5. Mann-Whitney U Test
The Mann-Whitney U test is a non-parametric test used to compare differences between two independent groups when the dependent variable is either ordinal or continuous, but not normally distributed.
Null Hypothesis (H₀): The distributions of both groups are the same.
Alternative Hypothesis (H₁): The distributions of the groups are different.
6. Wilcoxon Signed-Rank Test
The Wilcoxon Signed-Rank Test is a non-parametric test for comparing two paired samples to assess whether their population mean ranks differ.
Null Hypothesis (H₀): The median difference between pairs is zero.
Alternative Hypothesis (H₁): The median difference between pairs is not zero.
7. Kruskal-Wallis H Test
The Kruskal-Wallis H test is a non-parametric alternative to one-way ANOVA. It compares the medians of three or more independent groups.
Null Hypothesis (H₀): The medians of all groups are equal.
Alternative Hypothesis (H₁): At least one group median is different.
8. Fisher’s Exact Test
Fisher’s Exact Test is used to determine if there are nonrandom associations between two categorical variables, especially useful for small sample sizes.
Null Hypothesis (H₀): There is no association between the variables.
Alternative Hypothesis (H₁): There is an association between the variables.
9. Regression Analysis
Regression analysis is used to understand the relationship between a dependent variable and one or more independent variables. It can be used for prediction and to test hypotheses about relationships.
Null Hypothesis (H₀): There is no relationship between the dependent and independent variables.
Alternative Hypothesis (H₁): There is a relationship between the dependent and independent variables.
10. Logistic Regression
Logistic regression is used for predicting the probability of a binary outcome based on one or more predictor variables.
Null Hypothesis (H₀): The predictor variables have no effect on the outcome.
Alternative Hypothesis (H₁): The predictor variables have an effect on the outcome.
Conclusion
Understanding these key methods for hypothesis testing is essential for analyzing data and making informed decisions based on statistical evidence. Each method has its specific use cases, assumptions, and procedures, making them suitable for different types of data and research questions. By mastering these techniques, you can confidently apply the appropriate test to your data and draw valid conclusions from your analyses.To deepen knowledge and enhance skills in hypothesis testing and other data analytics techniques, consider exploring in a Data Analytics Training Course in Surat, Delhi, Ghaziabad, and many cities in india. A comprehensive training program will provide you with hands-on experience, expert guidance, and the tools you need to excel in the field of data analytics.
Comments