Understanding the Balance of Null and Alternative Hypotheses
- archi jain
- Oct 21, 2024
- 4 min read
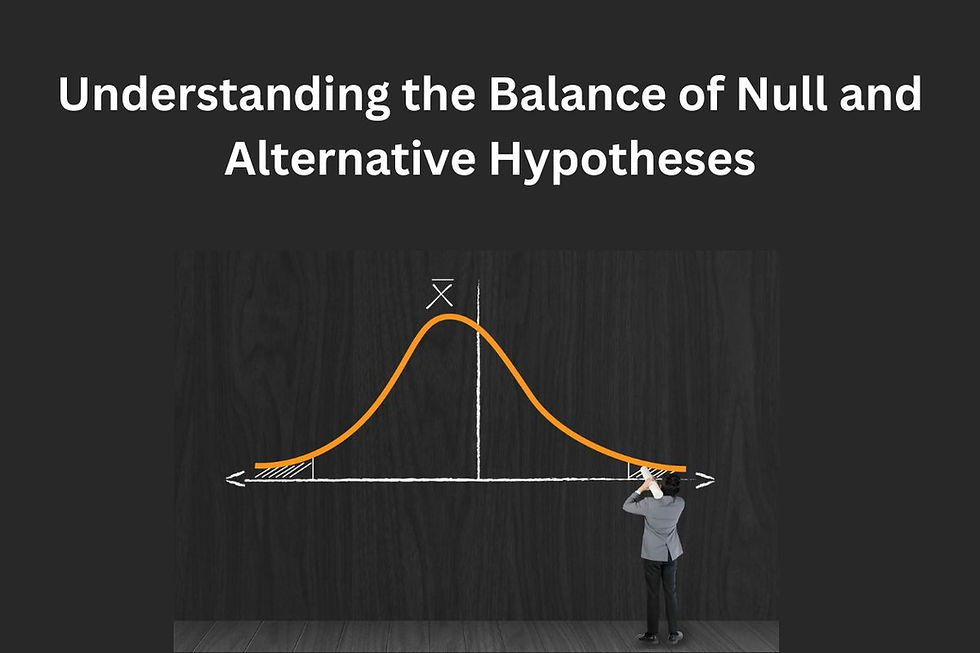
In statistics, hypotheses are crucial for drawing conclusions from data. Two main types of hypotheses are the null hypothesis (H0) and the alternative hypothesis (H1 or Ha). Understanding the balance between these two is essential for conducting effective research and interpreting results. Let’s explore what these hypotheses mean, how they work, and why they are important.
What Are Hypotheses?
A hypothesis is a statement that can be tested using statistical methods. It proposes a relationship between variables or suggests a particular outcome. In scientific research, hypotheses guide the data collection and analysis process.
Null Hypothesis (H0)
The null hypothesis is a statement that says there is no effect or difference. It’s like a starting point, assuming that any changes you see in your data are just due to random chance. For example, if you're testing a new medicine, the null hypothesis would say that the medicine doesn’t have any effect on patients compared to a sugar pill (placebo).
Alternative Hypothesis (H1 or Ha)
The alternative hypothesis is what you aim to support through your research. It represents a statement that indicates the presence of an effect or a difference. In the drug example, the alternative hypothesis would say that the drug does have an effect on patients.
Example
Let’s consider a study on a new educational program aimed at improving student performance:
Null Hypothesis (H0): The new educational program has no effect on student performance.
Alternative Hypothesis (H1): The new educational program improves student performance.
The Balance Between H0 and H1
Understanding the balance between these two hypotheses is crucial for a few reasons:
1. Establishing a Basis for Testing
The null hypothesis provides a baseline against which the alternative hypothesis can be tested. If we reject the null hypothesis, it suggests that the data provide sufficient evidence to support the alternative hypothesis. However, failing to reject the null does not prove that the null is true; it only suggests that there isn’t enough evidence against it.
2. Statistical Significance
Hypothesis testing involves calculating a p-value, which helps determine the statistical significance of the results. A p-value measures the probability of obtaining results at least as extreme as the observed results, assuming the null hypothesis is true.
If the p-value is less than a predetermined significance level (commonly 0.05), you reject the null hypothesis in favor of the alternative.
If the p-value is greater than the significance level, you fail to reject the null hypothesis.
3. Type I and Type II Errors
Understanding the balance between these hypotheses also involves recognizing the potential errors:
Type I Error (α): This occurs when you incorrectly reject the null hypothesis when it is actually true. For instance, concluding that the educational program improves performance when it does not.
Type II Error (β): This happens when you fail to reject the null hypothesis when it is false. For example, concluding that the program does not improve performance when it actually does.
Researchers often aim to minimize both types of errors, but there is usually a trade-off. Reducing the risk of a Type I error may increase the risk of a Type II error, and vice versa.
Designing a Study
When designing a study, researchers must carefully consider their hypotheses and the balance between them:
1. Clarity of Hypotheses
Clearly define the null and alternative hypotheses. Ambiguity can lead to misinterpretation of results.
2. Sample Size
Larger sample sizes generally provide more reliable results and help in making more accurate conclusions about the null and alternative hypotheses.
3. Choosing the Right Test
Select an appropriate statistical test based on the data and hypotheses. Tests include t-tests, chi-square tests, and ANOVA. Each test has its own assumptions and conditions.
4. Setting the Significance Level
Decide on the significance level (alpha). This threshold determines when you will reject the null hypothesis. The common choice is 0.05, but it can be adjusted based on the context of the study.
Practical Application
Let’s say researchers are testing whether a new teaching method enhances student engagement compared to traditional methods:
Define Hypotheses:
H0: The new teaching method does not increase student engagement.
H1: The new teaching method increases student engagement.
Collect Data: Gather engagement metrics from both teaching methods.
Statistical Test: Choose an appropriate test (e.g., t-test) to analyze the data.
Analyze Results:
Calculate the p-value.
If p < 0.05, reject H0 and conclude that the new method likely enhances engagement.
Report Findings: Clearly present the results, acknowledging the possibility of errors.
Conclusion
Understanding the balance between the null and alternative hypotheses is fundamental in statistics. It shapes how research is conducted and how results are interpreted. By clearly defining your hypotheses, designing your study thoughtfully, and being aware of potential errors, you can conduct robust research that contributes valuable insights to your field.
This balance is not just a statistical necessity but a crucial part of the scientific method, helping researchers explore the unknown and make informed conclusions based on empirical evidence. Whether in health, education, social sciences, or any other field, mastering this concept will enhance your ability to analyze data effectively. For those interested in furthering their skills in this area, consider enrolling in a Data Science course in Noida, Delhi, Gurgaon, and other locations in India to gain deeper insights and practical knowledge.
FAQs on Understanding the Balance of Null and Alternative Hypotheses
1. What is a null hypothesis (H0)?
The null hypothesis is a statement that there is no effect or no difference between groups in a study. It serves as the default assumption that any observed changes are due to random chance.
2. What is an alternative hypothesis (H1 or Ha)?
The alternative hypothesis is a statement that suggests there is an effect or a difference. It represents what researchers aim to support or prove through their studies.
3. Why are null and alternative hypotheses important?
They provide a framework for statistical testing. By comparing the two, researchers can draw conclusions about their data and determine whether to accept or reject the null hypothesis.
4. How do researchers determine whether to reject the null hypothesis?
Researchers use statistical tests to calculate a p-value, which measures the probability of obtaining results as extreme as those observed, assuming the null hypothesis is true. If the p-value is less than the predetermined significance level (usually 0.05), the null hypothesis is rejected.
Comments